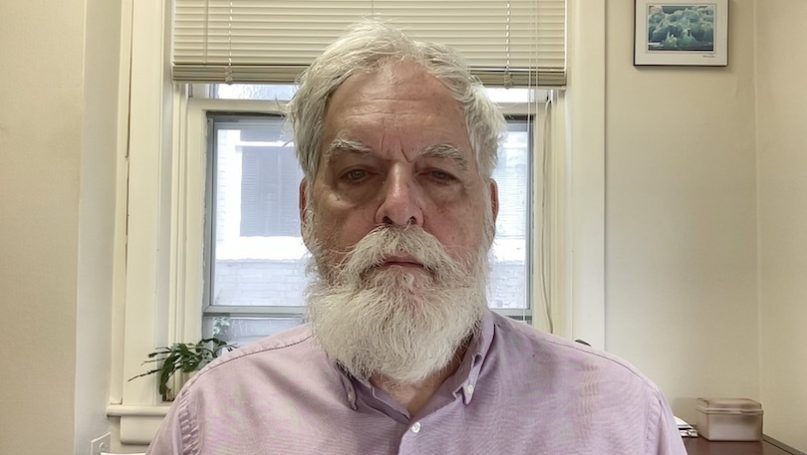
Philip Schrodt received his undergraduate and graduate degrees at Indiana University in the 1970s, combining formal studies in mathematics and political science with a lot of work at the university computer center, which housed a “supercomputer” with probably a fraction of the computing power of a Fitbit. He had a long academic career teaching international relations and political methodology with tenured positions at Northwestern University, the University of Kansas, and Pennsylvania State University, along with various visiting gigs, and extended field work in the Middle East, primarily the West Bank. Having published about 100 articles and after a couple dozen research grants, around 2010 he made the decision to leave academia, in the vain hope of providing a model for other Boomers to get out of the way (didn’t work), but at the very least to escape the omnipresence of 20-variable garbage can regression models and having to pretend these were important (that strategy worked). He spent the next ten years enjoyably and gainfully employed as a fulltime data scientist and software developer, primarily on projects related to forecasting political conflict, though is now slowly winding down those projects. He blogs at asecondmouse.org.
Where do you see the most exciting research/debates happening in your field?
Well, the extended rant I’m providing in response to this prompt has nothing, and everything, to do with the contemporary state of the field. As I complain endlessly, people in political science don’t realize that once you get outside academia you have no access to the literature because it is virtually all paywalled and can cost $35 just to look at a full abstract. “Your work might as well be buried under a pile of radioactive sludge In Antarctica.” Not everything is paywalled but so much of it is—even if you can get a few open articles, you can’t follow out the references—it’s not worth the trouble. That’s a completely different situation than computer science and data science, where everything important is accessible with a few clicks. Conferences? First, they are hugely expensive. And as for me, want to know how Harry Potter felt beneath that invisibility cloak? Go to an academic conference after the age of 55. Pre-COVID I was going to a number of invitation-only workshops, and those kept me in touch, and I still do a couple remotely, though I miss the individual interactions.
This lack of access has consequences: Who did the U.S. media turn to when trying to get a handle on Russia-Ukraine? John Mearsheimer! And specifically, his work in 1990 (“Why We Will Soon Miss The Cold War” and “Back to the Future: Instability in Europe After the Cold War“) about the implications of the end of the Soviet Union. Mearsheimer is a really smart guy and an excellent scholar, but that’s saying the past 30 years of IR scholarship is irrelevant beyond the academic community. Ouch! For that situation, you can mostly thank the paywalled journals which support the parasitic bureaucracies of professional organizations and “university” presses, and the deans, associate deans, assistant deans, and their endless layers of tenure committees who only look at paywalled journals when deciding on tenure.
Do the math: typically, about five years pass between getting an idea and getting it published in a top journal (after multiple revisions which typically make the work ever more boringly conventional), so a full decade passes before that idea itself is used in a publication. In computer science and data analytics, a really hot new method will be publicized, tested, and refined, and available in multiple open-source implementations in three to six months, as we are currently seeing with neural network innovations.
This is an admittedly extreme example but look at the Deepmind (a subsidiary of Alpha/Google) AlphaFold system—a large, specialized neural network—which solves the protein folding issue that has been at the absolute core of biochemistry for about 50 years, during which time about 100,000 proteins had been solved. AlphaFold is entered in a competition in June-2020, the spectacularly accurate results are described in Nature in June-2021 and 350,000 proteins had been solved, and by June-2022 it had solved 200-million protein sequences, essentially all known cases. And all of the results are available in an open-access database (see DeepMind, Washington Post, Nature etc.) More frivolously, the text-to-image systems such as DALL-E have gone from seemingly impossible to ubiquitous in mere months. Of course, there’s a not-insignificant part of the academic community for whom this is a feature, not a bug: they revel in irrelevance. But I’m with Francis Bacon—the philosopher, not the artist—and believe that the sort of work we are doing should benefit humankind. Or at least try to.
How has the way you understand the world changed over time, and what (or who) prompted the most significant shifts in your thinking?
Compared to graduate school and my early years in the field, I pay a lot more attention to history now, and try to read pretty widely, not just the same two or three works everyone reads or pretends to, like Thucydides (though Thucydides is pretty good). In the last decade or so I’ve paid a lot more attention to Chinese (and Asia more generally) history: these people were writing incredibly sophisticated philosophical treatises long before one saw work of comparable complexity in Europe (1000 years of elite illiteracy following the end of the western Roman Empire didn’t help: nothing of that magnitude occurs in Asia). I’ve also been reading a lot of early Buddhist writings—these can be reliably dated to about 2100 years ago, and many probably go back 2400 years or so—and from these, you see human psychology, both individual and collective, just has not changed that much (the buzzword I like is that it is “evergreen”). Finally, I’m reading more political anthropology—the late Marshall Sahlins, I’m a fanboy! And of course, James C. Scott: the Western ‘human nature’ we spend most of our time studying is an incredibly narrow sample of how humans can organize themselves. What/who was mostly due to fairly extended work, mostly with NGOs, in the Middle East, particularly Palestine thanks to Ibrahim Abu-Lughod, and some additional time in West Africa thanks to Leo Villalon.
To what extent is political and social behaviour predictable?
We’ve been the apex species on this planet for at least 50,000 years and apex species make their environments predictable. So, most of us most of the time through most of history (and pre-history) led a rather tranquil existence and died peacefully in the presence of someone who cares about us. As Sahlins points out, Hobbes (and for that matter, Rousseau and Bakunin) didn’t know the first thing about actual primitive societies and Hobbes instead inferred from his own highly structured society which was undergoing numerous socio-political transitional stresses. “Nasty, brutish, and short” was the result of political chaos in early modern England; real pre-modern societies, and generally the further away from centralized authority you can get, the better, are by most evidence usually a lot more pleasant.
And moving to the present, urbanized industrial societies are “self-domesticated”, where the average denizen just has to get to their lucrative if pointless corporate job and back home again using a massive system of public infrastructure, pick up their food at the QuikMart, and settle back into a self-imposed anxiety-producing regime of reading clickbait and outrage on social media. Yes, this urban industrial stability can break down in spectacular and catastrophic ways, as with WWII and now Russia-Ukraine, but, with essentially all four horsemen of the apocalypse—war, famine, disease, and [sudden] death—largely under control for the middle and elite classes everywhere in the world, we’re in the most predictable era in human existence.
What have been the most promising developments in accurately forecasting political and social behaviour in recent years?
Two major developments. First, the fact that systematic forecasting is now taken seriously: pre-COVID, Gregor Resich organized a couple conferences in Berlin—I characterized these as “the SunDance Film Festival of Conflict Forecasting”—and it was amazing to see the number of quantitative projects underway by governments and IGOs, though most were below the radar. The US multi-agency Political Instability Task Force (PITF) under the leadership of Jay Ulfelder was the initial proof-case of this, and PITF models have now been used in the US for almost two decades now, again, under the radar, but now they have been adopted internationally. The second is the proliferation of data on the web, both primary sources such as news reports, and long-term secondary sources such as Global Terrorism Database and Uppsala Conflict Data Project, as well as a couple relatively recent near-real-time data sets I’ll discuss below.
So far, ironically, new methods have not made a difference: PITF primarily uses logit models, which are embarrassingly effective. We certainly tried, individually and collectively, to find better alternatives, either in terms of models or new variables, but to date they just don’t seem to be there. But this could be changing: the new neural network transformer models are both sequence based (like the human cognitive structures of episodic memory that self-organize predictable systems) and—this was an amazing accident that has come as a surprise to everyone—exhibit a remarkable degree of social ‘common sense.’ That accident was due to the models being trained on the whole of Wikipedia, which was selected simply because it has a huge amount of generally coherent text in an assortment of languages, but, of course, much of that text is organized around narratives of individual biographies and historical socio-political behavior, so a great deal of that regularity ends up embedded in the models, which have billions of parameters (and are getting continually larger as they are useful in a very wide variety of commercial applications). These models and the software needed to process them are open, and a subscription to the Google Cloud hardware required to use them currently costs just $10/month (Google has excess capacity, it seems…), so I’m guessing we will see some major applications to conflict forecasting fairly soon.
In ‘Forecasting of Political Conflict: Right Data, not Big Data‘ you highlight the accuracy of data-driven political conflict forecasting is around 80-85%. What are the main challenges in relation to accurately predicting conflict?
I think that 85% ‘speed limit’, which is the same number we get from both the best computational models, whether statistical or machine learning, and from the human ‘superforecasters’ of Philip Tetlock’s Good Judgment Project (see Philip Tetlock and Dan Gardner Superforecasting: The Art and Science of Prediction) is pretty fundamental and I’ve got a list of about eight reasons—Tetlock has pretty much the same list, independently—as to why this is the case: the limit is due to effectively unpredictable things like natural deaths and disasters, systems exhibiting chaotic behavior (these can be very simple) and ‘rationally unpredictable’ behavior one sees, for example, in solutions to many zero-sum games. We’re dealing with an open system, not a closed deterministic one, and there are lots of decisions, big and small, individual and collective, that can plausibly go multiple ways: imagine world history had Zheng He’s massive fleet sailed into Lisbon’s harbor in 1420 rather than Columbus’s little ships accidentally encountering Hispaniola about a century later.
But, speaking of Tetlock, we’ve been in political systems where the typical policy ‘expert’ works at only 50% to 60% forecasting accuracy—this is the core result of Tetlock’s earlier groundbreaking Expert Political Judgment: How Good Is It? How Can We Know? Getting to 85% would be a huge improvement, provided this can be incorporated effectively into policy. Some places it has been, such as famine forecasting models (and, of course, hurricane forecasts) but so far it largely hasn’t: most policy makers would rather read Mearsheimer (and their ‘experts’ certainly don’t want to read Tetlock!). While on this topic, a recent independent study, with 164 co-authors from the Forecasting Collaborative at Waterloo University, Insights into accuracy of social scientists’ forecasts of societal change is generally consistent with Tetlock’s findings, this time with respect to social scientists, a wider variety of questions, and a baseline of very simple statistical models rather than just chance.
I think a frontier does exist for moving these systematic models to a much finer temporal and geographical scale. Superpower behavior excepted—Russia/Ukraine, US/Afghanistan, US/Iraq, China/Taiwan—violent conflict occurs at a sub-state scale (and for the most part, always has), so Nigeria, or even something as small as Israel is a really inappropriate unit of analysis for violent political conflict. Nigeria at present has three distinct foci of violent conflict: Boko Haram in the northeast, farmer-herder violence (farmer-herder conflict – imagine that! Didn’t Ibn-Khaldun have something to say about that ca. 1400 CE?) in the middle of the country, and a complex combination of oil-related piracy and long-standing ethnic disputes in the south. Israel, which is so small you could drive across it in less than a day except for the traffic jams, has four almost completely distinct conflicts in Gaza, the northern West Bank, the Lebanon frontier, and the Syrian frontier. So, we need to get way more specific in terms of geography.
Time is a little trickier, since most predictors of conflict in our existing models are slowly changing structural variables—state capacity variables such as GDP/capita and infant mortality rate—and historical ‘bad neighborhood’ indicators. In almost all of the models I’m familiar with, conflict probability accuracies don’t decline much as you go out further in time, which is very different from most physical systems (or election forecasts). But with the new near-real-time datasets, we will probably see a lot more experimentation at shorter time intervals.
As an example of what might be a “solvable” problem, we know that most sub-state conflicts have varying levels of intensity: at the very least, the involved parties, unless they have very significant and consistent outside support, need to re-arm, which also involves very significant expense, and it is also likely these periods would be safer and possibly more effective for international aid, and possibly negotiations (long ago there was a nascent literature on ‘ripeness’ for negotiation, though I think it was mostly hindsight and didn’t go anywhere). With a sufficient number of consistent sequences on these conflicts—there is no shortage of cases, but covering them can be very difficult—could we find regularities? Maybe, maybe not, but it isn’t crazy to try. But we just didn’t have this sort of finely grained data until recently.
With the advent of machine learning models that are so complex they are not entirely interpretable to humans, what kinds of challenges does this pose to using these more advanced models to drive policy and decision-making? Can these challenges be overcome?
Well—you expected this, right? —that horse—no, a total stampede of horses—left the barn long long ago, whenever editors of journals decided that whenever Reviewer #2 wanted another ‘control’ variable added to a regression, it had to be added, even though it was highly collinear with the existing independent variables and played havoc with the covariance matrix of the coefficient estimates. A regression model looks transparent—you are just multiplying coefficients by values—but how those coefficients were obtained is utterly opaque and, often as not, may be dependent on a particular numerical minimization algorithm in the case of logit, or round-off error in the inversion of a nearly singular matrix in conventional regression.
So how do we cope with this? Replication, both in the sense of exact replication, which has uncovered untold mistakes, honest and otherwise, but more importantly by thoroughly exploring the potential explanatory space relevant to various theories with multiple data sets, methods, and combinations of variables. Theodore Sturgeon’s Law: “90% of science fiction is crap? 90% of everything is crap!”: but we cope. In the academic literature, this is done slowly over the accumulation of many, many efforts, and the aforementioned unconscionable publication delays; in the policy community, where efforts can be more focused, there are existing techniques to systematically explore the model and data space, though some of these are fairly computationally expensive.
But on something of a side note, we need to pay more attention to the off-diagonal cases: the false positives and false negatives (or the large outliers in a continuous model). These are that gossipy little pest who hates their job and can’t wait to tell someone about it: anyone who has ever done an organizational review knows you learn far more from those people (ideally, several, telling more or less the same story: this is equivalent to triangulating the outliers in multiple models) than from those who say, “Hey, everything here is fine!” For example, after a decade or two or three of countless articles on multiple data sets, we can be pretty confident that democratic peace is solid except for those angels-dancing-on-pins exceptions like Finland in WWII. Or how Russia, not Poland, invaded Ukraine; or how China, not Japan or the Philippines, is sending missiles over Taiwan; and how the US invaded Iraq and Afghanistan, not Mexico and Canada.
The conflict forecasting community works a bit differently than academia, but remember it is a community: we mostly know each other and follow each other’s work. The various groups looking for something similar to the PITF models did, in fact, come up with largely similar results, and had someone found a really solid additional predictor, say political polarization, using a wag-the-dog theory, you can be sure that would have been rapidly disseminated in the community. Which, again, may well happen with the neural network models.
In your presentation Operational Choices in Generating Real Time Political Event Data you have highlighted that, for the first time in human history, we can develop and validate real-time measures of human behaviour and political information. What are the implications of such a development?
It’s still a little early to tell: we haven’t had fine-grained near-real-time data on a global scale until the last ten years, if that, when news sources went onto the web on a global basis, which occurred fairly quickly during 2005-2015, and then reliable near-real-time sources such as ACLED and ICEWS only became available in the last five years or so. Now we’re getting major projects using this sort of data—Uppsala/PRIO ViEWS is the most important one I know, and there’s more stuff going on in governments and IGOs—but it’s just beginning. The experience with COVID was a wake-up call for a number of people in the global community: in many instances basic data were not available, or were not of consistent quality, and that hindered both the monitoring and response. The World Bank, for example, is now investing $2.5-billion to improve data collection, particularly in countries with limited resources.
That said, I think this change will eventually be profound, but we will probably need at least a couple human generations to see this (that’s the rule-of-thumb for the effective adoption of new technologies such as steam, electricity, and computers in industry: I’ve seen little evidence the social sciences are any different, and as Max Planck observed, science advances one funeral at a time). I say probably because the best models are no better than the best human super forecasters, meaning that it is possible to get this sort of accuracy just by relying on well informed people (assuming they can get the sustained attention of the relevant policy makers), and I’m guessing some organizations and leaders had a good intuitive sense of who to listen to, so I may be unduly pessimistic about the accuracy of policy-relevant forecasts in the past.
But it’s really tough to get forecasts, particularly those with unwelcome implications, taken seriously, and notice that almost everyone we revere for their ancient political wisdom—Confucius, Sima Qian, Thucydides, Machiavelli, Hugo Grotius—were failed bureaucrats in their day, and countless more ended up forgotten in the mists of time.
But we can also see progress: macro-economic theory and its applications to policy have created a much more stable system in developed economies than existed in the first couple of centuries of industrial capitalism—’bank panics’ were once a regular occurrence—and I’d like to think we can improve in the international system. Even though a lot of things have ‘worked’, at least so far, in response to Russia’s 2022 invasion of Ukraine (they utterly failed for the 2014 invasion), I would hope we’d see some adjustments to international institutions as a response, particularly since we haven’t really modified these since the post-WWII era. Same on the economic side where tiny groups of elites—Lebanon, Sri Lanka—can essentially hijack a national economy and run it into the ground with complete impunity thanks to Western-created off-shore accounts.
You have stated that data generation methods and predictive models need to be transparent and open source. Why is this transparency so important? Do you believe that the firms and individuals involved in the political data science sphere are being transparent enough?
I’m only involved in the policy sector, so I’ll address that first, and more generally, I’ve written a fairly extensive piece on this here. I think the norm we should use are central banks, or at least the case I know from colleagues who have worked with them, the U.S. Federal Reserve. We don’t know exactly their models, and in addition—as we see with instability forecasts—models are only one input to policy, and the judgment of at least somewhat accountable human experts remains a major factor. But on the quantitative side, people have a pretty good idea of the sorts of data and the sorts of models that are being used, and much of this comes from a healthy interchange between long-term professionals and academics.
I wish we had something closer to this for instability forecasting, though thanks to PITF and some other projects like the U.S. Dept of Defense Minerva program, we have at least some. But for example, in the U.S. Defense Advanced Research Projects Agency (DARPA) Integrated Conflict Early Warning System (ICEWS) competition, the academics had virtually no input in two of the three projects (both lost the competition: I’d like to think this is not a coincidence), and in the long haul were absent even in the winning project as it moved to an operational phase. ICEWS started with long speeches from the DARPA program directors about how the sponsors hoped this would be a new phase of defense-academic cooperation and result in lots of refereed publications: none of that happened, and I believe only a single refereed publication came out of the entire $50-million effort (though much to its credit, a near-real-time data set did result after about ten years).
On the IR policy side, once you bring in the major defense contractors, the likes of Lockheed, Leidos, and BBN—and it is next to impossible to avoid this as the contracting requirements are so complex—every institutional norm is running against transparency, and no one in that sector was ever penalized for keeping things too confidential, even in the absence of formal classification. As I point out in the blog, some of this is needed—there are some truly bad actors out there—but applying the same confidentiality criteria intended to protect models of Russia and China to models of village-based militias in South Sudan doesn’t make sense.
As for the private sector, the record is mixed. Their internal models are far more invasive and worrisome than anything I’m aware that Western governments are doing. The utterly massive social media conglomerates make their money stirring up as much bad stuff as cleverly as they can: anger, greed, and delusion are their business model. Of course, this is certainly not the first time we’ve seen this (and ‘fakes’): the yellow journalism era about 150 years ago, using the then-new technologies of high-speed presses, cheap paper, and working-class literacy, had the same model, to a sometime ludicrous extent such as claims to have spotted a civilization on the Moon, and had serious consequences such as the Spanish-American War (the probably apocryphal telegraph from publisher William Randolph Hearst to artist Frederic Remington: “You furnish the pictures; I’ll furnish the war.”). And our current situation is orders of magnitude more intrusive.
Serious question: is the Chinese Great Firewall having more effect on politics by preventing people from seeing information, or do the Western social media, particularly Facebook, Google, and YouTube, have more influence by directing people to certain information? Particularly when much is viciously targeted to vulnerable individuals such as teenage girls worried about their body image or convincing people who have no jobs but plenty of military-grade firearms that massive conspiracies that may or may not involve alien lizards are responsible for every bad thing that happens?
In terms of the transparency of methods, there are some curious dynamics, probably mostly involving incentives for recruiting and retaining “talent” (and the large firms’ confidence in their dominance in hardware), which have led to the large companies—Meta/Facebook, Alphabet/Google, Microsoft, and Amazon—being quite open about advances in methodology, at least at the moment. So, there is a great deal of transparency on that side. For what it’s worth, for every time I’ve seen an industry or government application where my response is “Wow, cool, I wouldn’t have thought to do that!” I’ve seen about ten where I’m thinking “We tried that 10/20/50 years ago, and it doesn’t work.” Generally, I wish we had something more like the industry-academic exchange, which is nearly instantaneous, in computer science, or even economics. Between classification on the policy side, and publication delay and pay-walling on the academic side, and the lack of easy lateral entry on both, we just don’t see it.
What is the most important advice you could give to young scholars of International Politics?
Do significant field work in some place entirely unfamiliar, and for many readers, that would mean outside Europe and North America (though more North Americans also need to get over to Europe). You need to see how the world doesn’t conform to your expectations: spend a few days (or months) with a school teacher who lives in a one room hut illuminated with a single lamp in a small town but speaks six languages, including French, English, and Arabic, and can discuss global politics for hours.
If you are mostly qualitative, going deeply into Asian political history and philosophy (if you are Asian and already know all this, study in depth the emergence of the European state system during the early modern period. If you are not Asian and still think the Treaty of Westphalia is important, you also need to study the early modern period more seriously.) If you are mostly quantitative, learn a programming language in depth: Python would be my choice right now, but any general-purpose language will do, just do it in depth.
Do whatever you need to do to finish a dissertation reasonably quickly and get out on the job market, and ignore the advice of your dissertation committee who just want you to be a clone of themselves. Such cloning is impossible as the Boomers, at least in the U.S., in their quest to get out of the classroom and into proliferating layers of administrative positions which do nothing beyond calling meetings to create never-to-be-implemented ‘strategic plans’, have largely destroyed a once vibrant, public-regarding, affordable, and self-governing university system. So, if you can’t find an academic job, don’t think it’s your fault: the environment has profoundly changed over the last three decades. But the multiple skill sets required to design and implement a dissertation-scale project are both admirable and will take you far outside of academia: people wait too long to leave, chasing an utterly hopeless dream. Don’t make that mistake: It’s a magical world—go exploring!