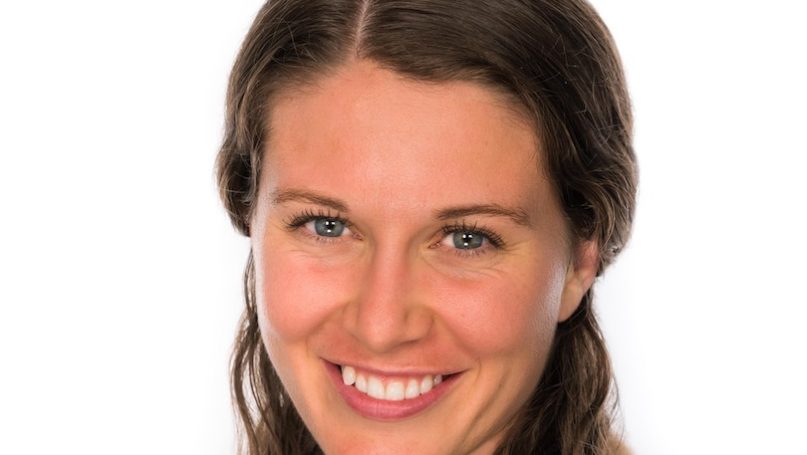
Katherine (Katie) Keith is currently an Assistant Professor of Computer Science at Williams College. Her research interests are at the intersection of natural language processing, computational social science, and causal inference. During 2021-2022, she was a Postdoctoral Young Investigator with the Semantic Scholar team at the Allen Institute for Artificial Intelligence. She graduated with a PhD from the College of Information and Computer Sciences at the University of Massachusetts Amherst where she was advised by Brendan O’Connor. She has been a co-organizer of the First Workshop on Causal Inference and NLP, a co-organizer of the 2022 NLP+CSS Workshop at EMNLP, a host of the podcast Diaries of Social Data Research, a co-organizer of the NLP+CSS 201 Online Tutorial Series, and a recipient of a Bloomberg Data Science PhD fellowship.
Where do you see the most exciting research/debates happening in your field?
I think the most exciting debate that has lasted for decades is the neuro-symbolic debate in artificial intelligence (AI). This refers to the debate of the most effective approach for AI to understand and interact with the world: through neural networks (deep learning) or symbolic reasoning. Neural approaches (deep learning) learn from data directly and can pick up patterns in data that have a lot of irregularities and edge cases, like language. On the other hand, symbolic reasoning, or the classical AI approach, involves predefined rules and logic for problem-solving, which is advantageous for tasks requiring explicit reasoning and understanding but can be brittle in the presence of irregularities. My prediction is that we’ll see a blend of these two in the future.
How has the way you understand the world changed over time, and what (or who) prompted the most significant shifts in your thinking?
I entered grad school believing that science was a “pure”, objective, neutral process of uncovering universal truths. Now, my perspective has shifted to seeing science as being much more of a social process. I have seen how individual’s values, biases, and social dynamics can and do influence scientific findings and their interpretation.
How has the rapid growth in methods and techniques for quantitative analysis affected how we measure human behaviour? Are algorithms playing an increasingly larger role in controlling human behaviour?
I’ve seen that this rapid growth has increased the need for computational techniques that scale. This has the advantage that we can have greater statistical power in our analyses, but this also has the disadvantage that anomalies and bugs in the data sets themselves are not as easily identified. I don’t believe algorithms control human behavior but I do believe we are enormously susceptible to them. I think we all have the responsibility to educate ourselves and others about their effects.
Why is transparency of large-scale algorithm audits important? When looking at YouTube’s demonetization algorithms, what are the difficulties in determining the association between a video’s topic and the characteristics of its demonization?
Transparency is important because as we say in our paper, “In recent years, changes to the monetization policies and the introduction of algorithmic systems for making monetization decisions have been a source of controversy and tension between content creators and the platform. There have been numerous accusations suggesting that the underlying monetization algorithms engage in preferential treatment of larger channels and effectively censor minority voices by demonetizing their content.” I think this is a question for policy makers (and one I don’t have an easy answer to) about navigating the tradeoffs between firm competitiveness and the protection of consumers and content creators.
Regarding YouTube, it’s not difficult to quantify the association between a video’s topic and the characteristics of its demonization, but it is difficult to pin down the mechanisms that generate it. As we say in our paper “Although we find instances of word-types occurring with high demonetization rates, the fact that there is no single keyword resulting in a 100% demonetization rate suggests that demonetization decisions are not made solely based on the presence of specific words in titles.” This suggests that there’s not an automatic keyword-based trigger in the algorithm, we had initially hypothesized might have been present.
In your paper on Causal Inference in NLP you highlighted that causality is emerging as increasingly important in NLP. What are the use cases of advancements in causality research in NLP?
In that paper, we highlighted two directions of causality and NLP. One was causality helping traditional NLP tasks, but the other direction is what I’m most excited about–NLP helping causal inference. We need to blend NLP methods into causal inference and this integration is not 100% straightforward. I recently wrote a paper that estimates the effects of peer review policies and we had to adjust for text as a confounding variable. I see many other domains like this in which blending NLP and causal inference could be helpful towards understanding causal relationships.
You were a crucial member of the team that created an advanced free online tutorial series aimed at teaching advanced NLP methods to the computational social sciences. What was the overarching ambition of this project?
Ian Stewart and I realized this kind of translational work—from NLP to the social science community—was really undervalued by our field. Somehow “novelty” is valued over “dissemination of knowledge.” This was frustrating to us and we both wanted to put in effort towards changing this trend. I think novel large language models, like ChatGPT, will only make this translational work more important.
How do you foresee current and future AI tools impacting international relations and politics in general? Will these tools ultimately end up being positive or negative?
My PhD focused on social measurement. I think AI tools—large language models in particular— will be just another tool in the toolbox for computational social scientists who study international relations and politics but by no means a panacea. A large chunk of my research agenda is highlighting when the “off-the-shelf” tools fail and need to be improved for valid downstream inference. I don’t have a prediction about the aggregate benefit or harm of these tools. My hope is, of course, that these technologies lead to overall benefit, but I believe this depends on the values of the humans who wield these technologies and not the technologies themselves.
What is the most important advice you could give to young scholars of International Politics?
Be willing and ready to adapt. We’re in an era of rapid technological progress and the methods used today may not be the ones used a few years from now.